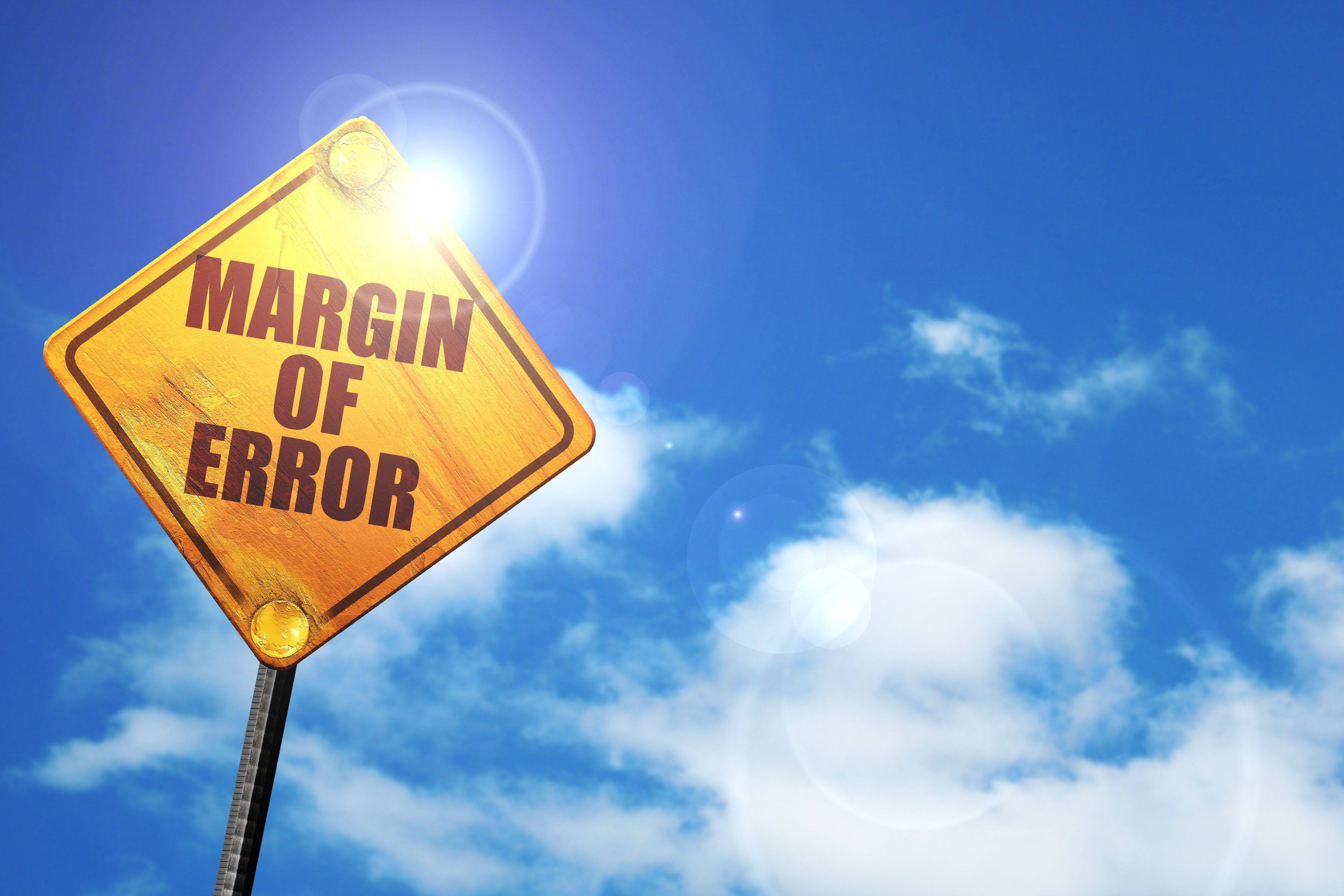
Margin of error plays a very important role in experiments and surveys that rely on statistics, indicating how reliable the survey and its results are. Any public survey, for example, takes a sample population from the whole population and then generalizes the results to the whole population. This approach invariably leads to a possibility of error because the whole of something can never be accurately described by a part of it. It is the margin of error that accounts for this inherent discrepancy. Margin of error tells you how many percentage points your results will differ from the real population value. For example, a 95% confidence interval with a 4 percent margin of error means that your statistic will be within 4 percentage points of the true value, 95% of the time. It goes without saying that the higher the margin of error, the less likely it is that the results of the survey are reliably accurate for the whole population.
Why is this relevant to cyber?
As we look to secure our networks and stave off cyber threats in the best and most efficient way possible, we must consider a few challenges that can make threat detection and hunting more difficult, and prone to errors and accidental oversights. If we can entertain the notion of a cyber margin of error (and its contributing factors), organizations can begin to minimize it and its effects.
What is widening the gap with the cyber margin of error?
- Diversity of attackers’ motivations and the difficulty of attribution
WannaCry has been classified as ransomware, motivated by the desire to make money. However, the NotPetya malware that quickly followed it, in June 2017, might very well have been a state-sponsored malware that attempted to disguise itself as ransomware, hoping to muddy its attribution in order to potentially delay investigations. These examples highlight the diversity of the attackers’ motivations and the difficulty (and even sometimes the impossibility) of attributing an attack. Lack of contextual awareness further erodes the possibility of minimizing the margin. - Early detection and mitigation of attacks
Since zero risk cannot ever exist, the early detection and mitigation of attacks is as important as the attempt to reduce the risk of successful attacks. Unfortunately, there probably will always be vulnerabilities in our systems and networks — despite increasingly efficient preventive security mechanisms — that make faster detection crucial. It is no longer sufficient to identify an attack during the attack; instead, we must strive to identify attacks even earlier … as early as during the staging phase. - Complex networks, distributed infrastructure and remote workforce
Remote workforce is growing in numbers and is mostly here to stay. The remote workforce, growing network footprint, and distributed infrastructure cannot be entirely secured through human intervention alone. Increasing the number of eyeballs to monitor the environment using the same old tools and frameworks is proving to be ineffective. Automated correlation of contextual information with alerts and events minimizes the mean time to detect, assess, and resolve across a massive footprint of users spread across complex networks and large infrastructures.
What needs to change?
The SIEM traditionally has been considered the top of the pyramid, as it is the only one in the triad to be able to ingest and analyze everything. Supporting the SIEM, you have the endpoint detection response (EDR) and network detection and response (NDR) present in the SOC triad. Traditional EDR and NDR vendors seem to subscribe to the notion of the SIEM being the all-seeing eye. While SIEMs do see across the vast threat landscape, can they still see everything when adversaries are constantly changing and camouflaging their TTPs?
It might be time to rethink the SOC visibility triad. Let’s think of the triad as an equilateral (pun intended) concept, with all three players holding up the three corners in a dynamic, interconnected system of networks. In this model, each strengthens each other, and forms the strongest cyber defense posture possible, collectively minimizing the cyber margin of error. It is a win-win-win scenario.
How does the IronDefense platform help to minimize the margin of error?
It’s understandable that organizations looking to secure their enterprise have long-treated log-based detection as a SIEM-only function. IronDefense is challenging and changing that notion. Here at IronNet, we treat security analytics, operational analytics and threat detection as a unified outcome, based on our analysis of both packet and log data. After all, any mature cyber security program marries the two approaches into one. That is why we are simply adopting this convergence into a native product capability. In short, IronDefense seeks to merge the insights gained from both packet analysis and log analysis. This combined capability allows us to detect and analyze at every step of the threat cycle, thereby minimizing the cyber margin of error through each of these three stages.
- Early Threat Warning
IronDefense not only identifies traditional Indicators of Compromise (IoCs) and known threats but also weeds out early indicators preceding an attack. It is what we have come to call “Indicators of Behavior” (IoB) across users, hosts, applications, and the network. Behavioral analysis allows us to identify potential attempts in the staging of an attack, either by an insider or an external actor. The IronDefense platform allows hunters to investigate and triage potentially suspicious behavior, scoring it as benign or dangerous enough to warrant further scrutiny and close monitoring. - Attack Warning
Once we identify the IoB(s), our platform proceeds to enrich and correlate the behavioral indicators with contextual information gleaned via manual hunting or automated generation of insights, risk assessment, and dark-data (data unique to the organization). At this stage, we can confidently identify the evolution of the malicious behavior into potential “Indicators of Attack” IoA(s), which are effectively mapped to the MITRE ATT&CK framework. The IronDefense platform then provides organizations with recommended actions to respond to this potential attack. - Breach
“Indicators of Compromise” are basically generated from the observed Indicators of Attack. We combine the context of the attack along with external/internal threat intel to confirm the nature of compromise. Ideally, with the IronDefense platform, we hope to help our customers catch the threat early on in the kill chain, well before the threat evolves into an expensive and damaging breach.
A contextually-aware analytic platform
IronNet’s cyber analytics see threats around the corner before they rapidly advance to the attack stages of the MITRE ATT&CK framework. When you add Collective Defense (that is, threat sharing in real time) to this ML-driven approach to cyber security, we all really do broaden our shared visibility across the threat landscape — making that margin of error as small as possible.