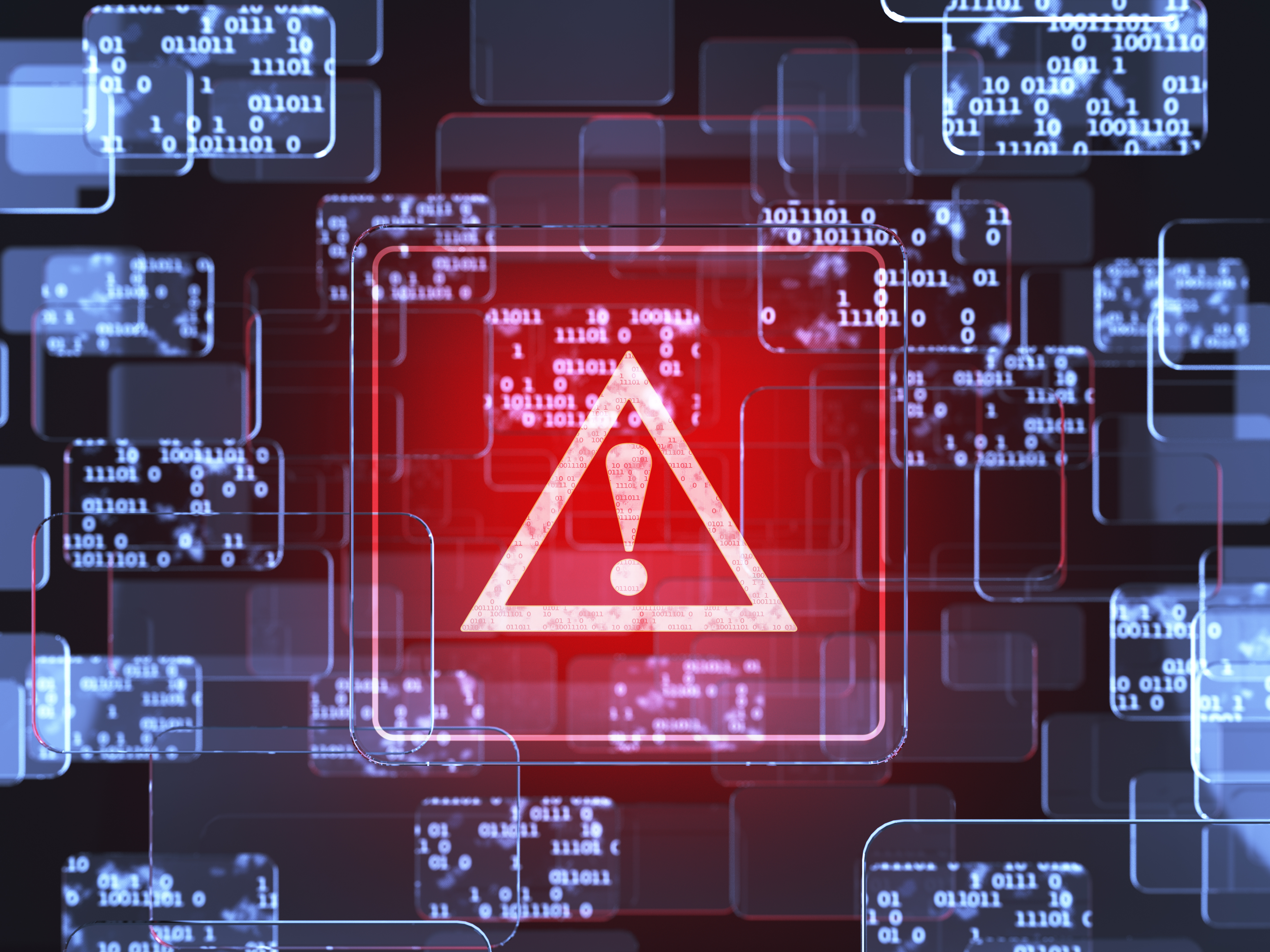
Editor’s Note: This blog entry was originally published on August 13, 2020, and was updated on September 7, 2022.
Merriam-Webster defines an expert system as “computer software that attempts to mimic the reasoning of a human specialist.” At first glance, that seems like a pretty broad definition that could be applied to nearly any operation for which a computer is responsible, but we believe the key word here is "specialist.”
Even the best-trained artificial intelligence (AI) model derives its value from the vast experiences and insights of human specialists. In fact, it is human insight combined with machine insight that offers a way to combat the “alert fatigue” known to most security operations center (SOC) analysts.
At IronNet, we look to specialists to solve this problem. Specifically, IronDefense, our Network Detection and Response (NDR) solution, takes the intellect of our highly qualified nation-state offensive and defensive computer network operation specialists (a.k.a., threat hunters) and packages it with anomaly and behavioral analytics skillfully crafted by our data scientists to produce the most relevant and effective prioritized alerts.
When we sought to develop behavioral analytics focused on network traffic, we did so for three important reasons. We knew that:
- Adversaries require network communications to perform the majority of their desired actions.
- There are gaps in endpoint detection due to the ability for malware to evade detection on the endpoint.
- Taking a passive approach to analysis would allow operation over the data without compromising performance or hindering business operations, unlike a firewall or intrusion detection system (IDS).
As it turns out, there is a prevalent problem with behavioral analytics: the amount of false positives this approach produces. The reason for what essentially amounts to “alert fatigue” is straightforward: adversaries that are attempting to attack our customers are doing so using similar behaviors to what is occurring on the networks as part of their normal business operations.
Adversaries know what typical network behavior looks like, and they seek essentially to hide within it. From our offensive experience, we knew how to succeed as an attacker.
From our defensive experience, we knew better solutions were needed. Behavioral analytics alone wasn’t getting the job done. This is where the specialist part of the equation is so critical.
Where human expertise makes the difference with threat detection
It’s easy to find examples of behaviors that could be malicious but are not always bad. Triggering alerts on these suspicious traffic patterns too often results in false positives, distraction, and alert fatigue.
Let’s take beaconing as an example. In this case, the behavioral analytic is looking for repeated, structured network requests that occur at a given frequency within a certain duration of time.
Innocuous beaconing |
Beaconing attack |
Calls to public facing infrastructure, giving information about the application such as version number & device type |
Calls to attacker command and control server, confirming that the malware was installed successfully, sending location & device type |
Calls out to trusted software developer to retrieve application updates |
Establishes communications with the command and control server to receive & execute malicious actions |
Software updates often eliminate security vulnerabilities |
Provides status and pattern of life information of both the malware and infected device |
Immediately, you can see the issue. Beaconing detection analytics are also likely to detect software update requests. This isn’t good enough to be useful.
IronNet built a robust testing platform to prove that our analytics could detect known malware and known malware techniques using only their behaviors, without any signatures in play. But we found that our analytics were also detecting benign activity.
At this point, we needed a way to refine our results such that we were definitively detecting only bad behaviors and not simply alerting on behaviors that could potentially be bad.
Tackling false positives to combat alert fatigue
So what about tackling false positives? Here are the three approaches we have taken:
1. Safe listing
Our first approach at addressing the false positives was safe listing. This approach works and is still an important part of our product offering; however, it does have flaws. Sophisticated adversaries know how to abuse safe or trusted lists by leveraging services that are typically believed to be benign, and thus are ignored when they show up as detected threats.
Additionally, what is approved as normal in one customer environment, may not be normal for a different customer. Further, there are many services and browsing activities running in an enterprise environment that are benign but will never be put on a safe list, simply because there are far too many to keep up with.
2. Threat intelligence
The second approach to reducing the false positives was to use threat intelligence. This method wasn’t going to help us find sophisticated unknown threats, but it does help to indicate when a suspicious behavior has leveraged indicators of compromise (IOCs) that have been previously used in a cyber attack.
This was effective and enabled us to deliver higher fidelity results to our customers. Our system was smart, however, we needed even more… we needed to push a little harder.
3. Expert insights
We needed a way to take the network anomalies and behaviors that our analytics had detected and prioritize the most likely to be malicious findings, letting them bubble to the top of the SOC’s list.
Safe listing and threat intelligence eliminated some of the chaff based on experience and observation, but neither technique was helping us find new and unknown bad activity.
The practice of finding the unknown is not about delineating between known good and known bad. It’s about operating in the uncertain, in turn rapidly drawing on expert insights to analyze and assess whether the activity is good or bad. This is what led to the development of the IronDefense solution.
Winning with threat hunting expertise on your side
IronNet’s specialist team has a lot of experience hunting to identify threats. Our hunt operations start with either threat intelligence or qualified leads discovered in the network by our NDR solution IronDefense.
We studied how our team operated, thinking about ways to make their job easier so that they didn’t have to comb through so many alerts that resulted in false positives. At the same time, we realized a few key things.
First, scaling is a huge challenge for cybersecurity. There is precious little talent capable of turning an unknown detection into a known bad discovery.
Second, the manually intensive process of hunting threats takes a significant amount of manual labor.
Third, alert fatigue is real. Focusing on real threats is essential.
Insert automation 101. This is where AI and machine learning (ML) combine with human skill to better detect cyber threats.
Enriching automated alerts with IronDefense
Our customers’ SOC analysts leverage their knowledge to assess whether the anomalies and behaviors found in their systems are more likely to be malicious or benign.
IronDefense was developed to operate over the anomalies and behaviors detected from the network traffic, automatically enrich them using numerous external and internal sources, and, within their context, assign the behaviors scores to indicate if they were more likely related to something malicious or something benign. The most likely malicious threats would bubble right to the top.
See how IronNet helps alleviate alert fatigue in the video below.
Aided by AI and ML, our experts continually improve upon the alert prioritization within IronDefense. Further, combining IronDefense with our IronDome solution results in the robust IronNet Collective DefenseSM platform that delivers threat knowledge and attack intelligence across industries at machine speed.
Attack intelligence is threat intelligence taken a step further - it’s timely, relevant, and actionable. It allows analysts to find and address threats before threat actors complete their goal.
By pairing behavioral analytics with Collective Defense, your organization can collaborate anonymously with others across industries and sectors to stay ahead of evolving threats through real-time threat sharing — and make alert fatigue a thing of the past.